Introduction to machine learning: what is it and applications
Maybe you have noticed that tech-websites are mentioning quite often something called "machine learning". Beyond what you can imagine, we can tell you that every day you use technology based on this technology. And yes, as the name implies, it is the automatic learning performed by computers to accomplish tasks using the experience they get from the data analysis. According to experts, in a not so distant future, this technique could make machines behave like us. In this introduction to machine learning, we show you what this fascinating subset of artificial intelligence is about.
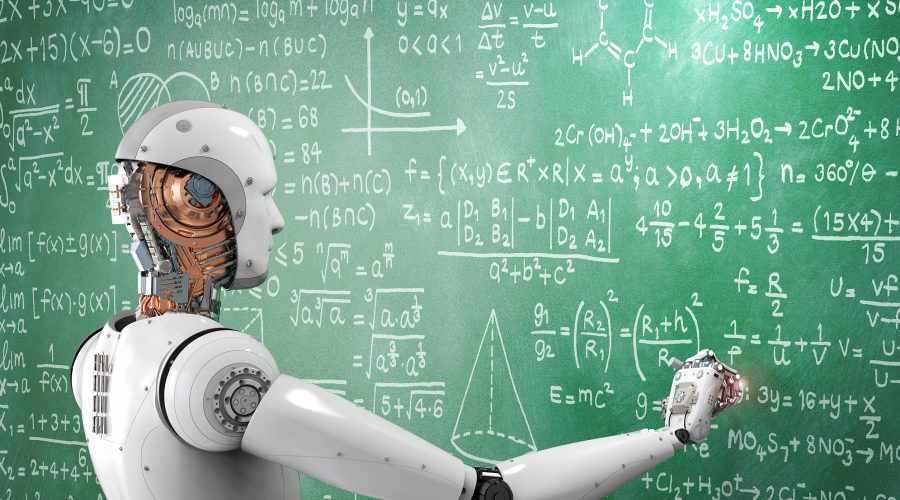
Introduction to machine learning: what is it?
Computers are evolving very fast and machine learning is the biggest probe. Why? Because machines started being programmed to learn from the experience resulting from data analysis just like humans do. This means that they could become autonomous taking their own decisions as machine learning keep evolving.
To exemplify it, think of a little boy who started learning what the world is about. His parents teach him to not play with the fire. However, the child does not know what fire can cause to him until he feels the pain of a burn.
From this experience, the child has learned that he should not play with fire. Now he will make wiser decisions to be careful when he gets close to any fire source.
The same learning process is what scientist want to achieve in computers through the machine learning technique.
So what is it exactly? As a simple introduction to machine learning, we can say that is a data analysis technique that teaches computers to decide according to experience. Just as people and animals do.
To achieve this, the machine is loaded with automatic learning algorithms that use calculation methods to collect information, learn from it and process it.
As these algorithms collect more information, the ability of the machine to make appropriate decisions improves and the error rates are reduced.
We must take into account that machine learning is not a conventional software application, but a system that can code itself by developing its own instructions from samples.
Machine learning in daily life
Although machine learning introduction still needs to go a long way until it can be compared to a human brain, we already use technology based on this technique. For instance Siri, image recognition systems, or even your email account.
In Siri’s case, you may notice that its voice recognition gets better as you keep using it. Siri begins to register with more precision the patterns of your voice as you “teach” it to do so. After a while, Siri’s errors to recognize your instructions decrease. This is thanks to the machine learning technique.
Voice recognition like Siri is an example of Machine Learning
A similar case is your email. In order to detect spam and regular emails more effectively, your email client needs to learn from the actions you take when using it.
For example, if you move an email from the spam folder to your inbox, the system will learn that emails from that specific address should be placed in the inbox and not in the spam on the following occasions.
As we mentioned before, in order for systems based on machine learning to reach high accuracy, they need to learn from the data they collect constantly.
The previous examples illustrate some of the simplest applications of machine learning introduction. However, in the field of science, machine learning helps doctors to detect tumors, decipher DNA sequences or develop medicines.
Do you know that...?
In Master Internet besides renting a cloud you can lease a more affordable virtual server (VPS). VPS servers technologically run on different hardware but they provide the same flexibility and high performance. On top of that, you can profit from a very fast set-up of the service.
Machine learning techniques
Machine learning has two operational models: supervised and unsupervised. The supervised model uses input and output data to make predictions. The unsupervised model tries to find hidden patterns in the information it receives. Let’s see them in detail.
Supervised learning
The supervised learning technique is effective to perform predictions according to a series of data or tests that the machine has previously collected. This data helps it to generate a reasonable model to solve uncertainty.
Let´s make it simple. Think of a doctor who treats a patient with heart problems. The doctor wants to know what are the chances for his patient to have a heart attack in the next six months.
The doctor gives the machine the data of his patient. Then, the machine compares this data with the data of similar patients who have had heart attacks. The machine takes into account physical aspects such as height, weight, age etc, of the current patient and the previous ones.
After it processes all the information, the machine can predict the chances of the patient to have a heart attack. Then the doctor knows what treatment will apply.
For the operation of supervised learning, two techniques are required: classification and regression. The classification technique is responsible for organizing the incoming data into categories, for example, if a cell is carcinogenic or not.
On the other hand, the regression technique is responsible for predicting continuous responses such as weather changes or electricity savings taking into account previous consumption.
Unsupervised learning
Unsupervised learning is based on a technique called clustering which is used to find hidden patterns in the data.
In other words, this technique is useful when you do not know what you are looking for. In this case, you can train the machine to find or track what you are looking for like a hound sniffing a prey.
The unsupervised learning technique can identify specific patterns within huge amounts of data. Something that a human could hardly achieve with such speed and efficiency.
Some applications of unsupervised learning are used by banks to detect fraud or by antivirus to find potential risks in the network.
Machine learning´s basic process
Conclusion
As we have explained in our machine learning introduction, this technique is based on the use of algorithms. However, there are dozens of algorithms to achieve different objectives through machine learning.
To select the right algorithm is important to know the nature of the data you are going to work with, and the results that you expect.
Either way, it will always be necessary to venture into experimenting to find the right algorithm.